Q&A: Machine learning, mathematical modeling used as tools to predict suicide ideation
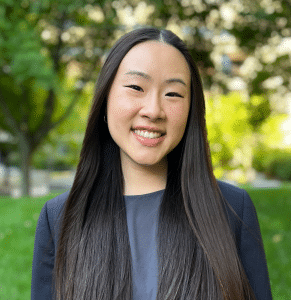
Modern psychology may be about to experience a sea change. While the practice has certainly evolved over the past 100 years, the introduction of machine learning, a form of artificial intelligence that uses large data sets to look at human behavior, may vastly alter the way mental illness is understood and treated.
At Yale University, Shirley Wang, Ph.D., who joined the psychology department in July as an assistant professor, is using machine learning and mathematical modeling to attempt to predict suicidal ideation and attempts. While each person’s experiences are unique, the goal is to use these tools to boil mental illness down to a purer science, almost a mathematical equation.
Speaking with New England Psychologist’s Catherine Robertson-Souter, Wang explained how mathematical modeling and machine learning can be helpful tools in predicting and treating suicide and other mental illness and how they may change the future of psychology.
First, can you explain a bit about the use of machine learning and mathematical modeling?
I am interested in improving our understanding of why people become suicidal and predicting when and for whom suicidal behaviors emerge. So, with machine learning, we take large amounts of data from things like smartphones, wearable bio sensors or electronic health records, and use that data in different algorithms to try and build prediction models that can say that, in this group of patients, this person is at highest risk of suicide. Or for this patient, this is when they might be at risk.
In other areas of science, theories are expressed as mathematical models, force equals mass times acceleration for instance. In psychology and mental health, we do not have anything like that. We have a lot of theories that are all very vague and very verbal. For instance, hopelessness relates to suicidal thoughts. Okay, but how and when, and why? What does that actually mean? So, that is what I am trying to do with the mathematical modeling, formalize our psychological theories as mathematical models.
What does that look like? How do you turn thoughts or behavior into a mathematical formula?
We recently wrote a paper that is not published yet, where we tried to take the first step at building a mathematical model of suicidal thoughts using factors like stressors in the environment or life events, emotions, the urge to escape negative emotions, all things that are typically present across suicide theories.
Then we used something called differential equations to express each of those model components in mathematical terms. Then we used that to simulate data and different cases, someone who has a lot of stress in their life and really does not cope well with negative emotions, for instance. What do our models say is going to happen over time?
How do you turn something inherently subjective like their level of stress or ability to cope into math? Is it based on bio markers or self-reporting?
Well, first we are working to formalize the relationship between the variables, but the variables themselves are still sort of ambiguous. We are hoping to eventually formalize those components yet still leave them vague enough so that they can be personalized.
What is the next step after a model of suicidal thoughts?
The next step is to try and model the transition from thinking about suicide to attempting suicide. There are a number of challenges there. Another thing I am working on is a smartphone monitoring study to incorporate different environmental contextual features to measure and include in our models.
What have you learned so far in your work?
That this is all immensely complex.
Historically, I think psychologists have approached studying and treating mental disorders as complicated rather than complex systems. ‘The problem is emotion regulation or cognitive rumination or under activation of the amygdala.’ We focus on one component at a time and isolate one factor at a time.
The thing is, we all have this sense that it can’t be boiled down to just one factor at a time or the interaction between two factors at a time. But the ways that we have studied it have been misaligned with this clinical intuition.
It’s really hard for us as humans to understand the dynamics of a complex system. So, I am trying to align those theories more by using things like machine learning and mathematical modeling to model the complexity.
Will creating these models of a complex system be useful to eventually provide treatment?
If we build a mathematical model that is robust, then maybe we could take one person and basically create a computer simulation that represents the symptoms that they are experiencing.
Then we could start to intervene on that simulated model and look at what are the downstream consequences. It’s not just an A causes B relationship. I hope that this can help with personalizing interventions to a point where we could say maybe for this person starting with CBT is going to work better but for this person starting with medication or starting with DBT will work better.
Another piece of your work is in looking at the disparities in health and mental health care for marginalized groups. How do you hope that this type of modeling will highlight and change the ways people are getting health care?
Black American youth, Indigenous Native American populations, and LGBTQ+ populations have some of the highest suicide rates. And we know that there are lots of health care disparities for people from racial and ethnic minority groups because of the systemic biases within healthcare.
Now that we are taking in all this data and trying to build models with it, we have to be aware that there is so much bias already in this data. If we are going to rely on machine learning models, we do not want that bias to be further baked in.
One thing we can do is to consider adding environmental triggers, experiences of micro-aggressions or racism in diagnosis and prediction. All of those affect mental health and they are very rarely included in our models.
Looking specifically at those populations and how we can avoid further stigmatization through our models is something that I feel very passionately about.
Working with college students, do you hope to understand from a mathematical point of view, why this age group is more at risk with suicide and eating disorders?
That’s exactly the type of complexity that I’m hoping to capture and model in looking at these things across development, especially in really critical periods, like adolescence and young adulthood.
Early emerging adulthood is such an important time when we think about things like body image disorders, eating disorders, self-harm. Talk about complexity. There are changes in your social group, your physical location, your daily activities, and the politics in the world around you and everything is changing at a rapid rate. It’s actually a perfect age group to focus on when trying to do these types of models.
Why is this work important?
Suicide is obviously one of the most devastating mental health outcomes and eating disorders carry some of the highest mortality rates of any psychiatric diagnosis. Being trained as a clinician myself and seeing the real-world impact of what it’s like to live with suicidal thoughts or what it’s like to struggle with an eating disorder, really drives home the impact of why this research matters. And then you think of the scale and the 800,000 people each year that are dying by suicide, it really highlights how important this work is.